Improving Care with Lessons from Search Engine Technology
Contributed Commentary By John Showalter
January 16, 2020 | Type “Fast Food” into Google, and without scrolling you’ll likely find the answers to any fast food-related question you could possibly want to know. A map displays nearby restaurants categorized by cuisine, price, distance, and hours open. Depending on where you are, there might even be reviews to help you find the best option in your area. Considering the billions of search results that could appear, how does Google know to show you exactly what you’re looking for?
Google isn’t just scanning the internet for search keywords—it guesses what you actually want to know based on your personal data. Depending on where you are or what you’ve previously searched, Google will tailor the results it shows to what it thinks you were looking for. The artificial intelligence (AI) can also easily identify when a search inquiry is misspelled or a word is left out and recommend what the user intended to search with consistent accuracy.
How the algorithm actually works is a closely guarded company secret, but Google isn’t the only one using data to understand user intent. In fact, intent-driven search is quite ubiquitous among search engines and other tech platforms such as Amazon. The technology works reliably, having matured over the last 20 years with the help of an army of Silicon Valley data scientists. But other industries — particularly healthcare — have been slow to apply the same cognitive science.
Healthcare has long been a few steps behind the digital technology curve. Electronic Health Records (EHR) didn’t gain widespread adoption until after the HITECH Act of 2009, when the federal government provided financial incentives to promote EHR adoption. That’s nearly 15 years after the internet was commercialized in 1995. And in many ways, using EHR today is a lot like using the internet in the late 90’s: a far cry from the seamless user experience of contemporary internet browsers like Chrome or Firefox.
Along those lines, a recent study conducted by the Mayo Clinic found that clinicians give the EHR user experience an “F” rating with a System Usability Scale (SUS) score of 45.9. For context, Google search has a SUS score of 93. Perhaps not surprisingly, the same study found a strong link between EHR usability and clinician burnout.
The user interface may be part of the problem, but alert fatigue is far more concerning. EHR systems bombard doctors and nurses with alerts, intended to point them to patients in need. Clinicians may receive hundreds of these alerts daily. But as many 80-99% of these alerts are false alarms or clinically insignificant. Clinicians quickly learn to ignore these alerts—at a potentially grave cost to patient safety and quality outcomes.
To reduce alert fatigue, EHR systems and the providers using them should take a cue from search engines. Google and others figured out long ago how to apply AI to filter search results and show users the most relevant results first. AI can and should be applied to filter clinical alerts in a similar way, screening out insignificant alerts and ensuring clinicians see only alerts that help them provide better care for their patients.
To be useful for clinicians, alerts must be actionable. Alerts that come only after a patient is harmed or imminently facing harm are of little value, coming too late for clinicians to take preventative action. Alerts without recommendations for clinical interventions are also of limited value, as clinicians are left to figure it out themselves. To be actionable, alerts should not only provide insight on a patient’s risk of future harm, but also insight on what clinicians can do to address that risk and prevent harm.
Much like with internet search results, data is key to making clinical alerts more actionable. And patient medical history isn’t the only relevant data; to fully account for a patient’s risk factors, AI should also account for behavioral factors and social determinants of health including socioeconomic status, access to quality nutrition, medical literacy, and environmental factors including weather and air quality. And, of course, when processing thousands of data points per patient, maintaining patient privacy should be a top concern for AI developers.
By coupling the analysis of patient data with an analysis of medical literature, AI also facilitates more personalized treatment, recommending interventions clinically validated to effectively treat each individual's unique profile. These personalized treatment recommendations are a powerful way to help clinicians take action and improve outcomes without overwhelming them with bothersome alerts.
For clinicians using EHR, finding the critical information they need to help their patients is a lot like the early days of the internet, when finding the information you needed was a frustrating process that required browsing through dozens of pages. But the stakes are much higher than where to find a good burger—EHR frustration and alert fatigue are major sources of physician burnout. Much as Google’s intent-driven search helped fulfill the potential of the internet, AI tools can help clinicians make the most of their EHR by directing them to exactly what they need to know to make the right decisions for their patients.
Dr. John Showalter, MD is the Chief Product Officer at Jvion and a board certified internal medicine physician specializing in clinical informatics. He is passionate about quality improvement and the application of AI to improve patient outcomes, and is dedicated to ensuring the Jvion Machine drives value and improves lives. He can be reached at john.showalter@jvion.com
Leave a comment
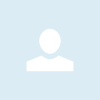
